How ML Systems Can Unlock or Suffocate Your Innovation
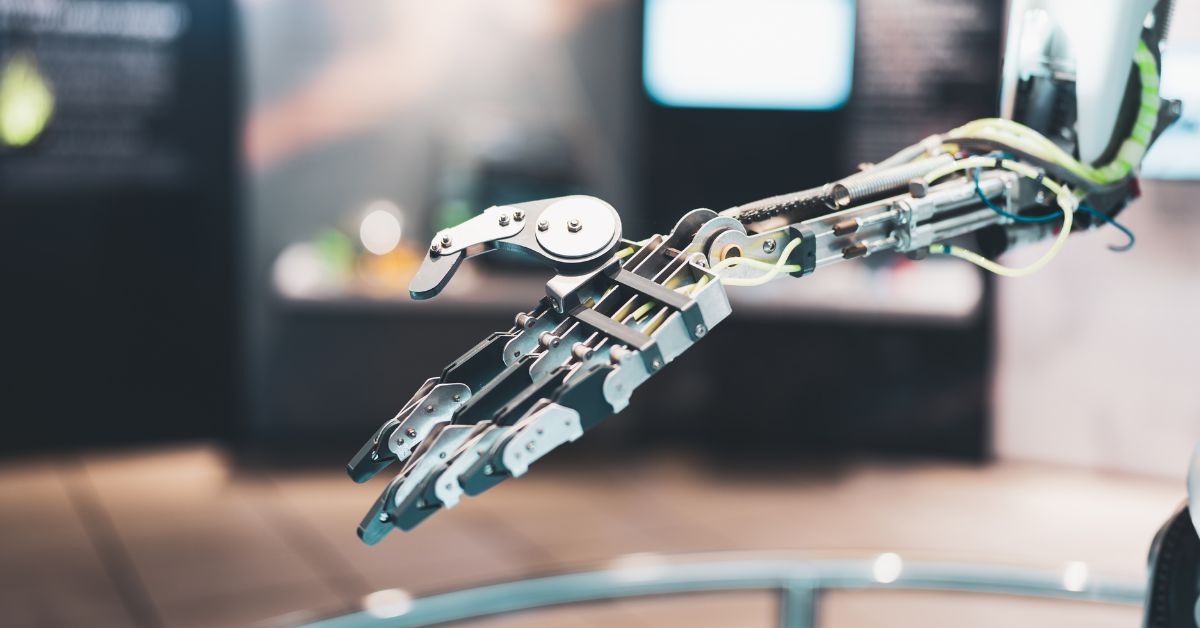
In the fast-paced world of technology, ML systems (machine learning systems) are playing increasingly critical roles across various industries. These advanced computing solutions have the potential to transform operations, drive efficiency, and foster innovation within businesses. By automating repetitive tasks, they enable staff to focus on more strategic initiatives. Moreover, they can process and analyze data much faster than humanly possible, leading to quicker insights and the ability to act on those insights in real-time. This creates a dynamic environment where decision-making is informed by up-to-the-minute data, driving businesses forward in a competitive marketplace.
The impact of machine learning systems extends beyond mere automation. They are integral to identifying trends and anomalies that would be nearly impossible for humans to detect without significant time investment. For example, ML systems can monitor customer behavior to provide personalized experiences, anticipate future needs, and identify areas where businesses can offer enhanced services. Additionally, they improve operational efficiencies by optimizing logistics, reducing downtime, and predicting future demands. In essence, ML systems not only streamline current practices but also pave the way for creating new business models and revenue streams.
Furthermore, as these technologies continue to advance, they are opening up possibilities in areas that were once considered beyond the reach of automation. Complex problem-solving and creative thinking are progressively becoming the domain of ML systems, as they learn from successes and failures and continually refine their algorithms. They’re enabling businesses to explore new possibilities, challenge established norms, and rethink how they operate in the digital age. While adoption of machine learning systems requires thoughtful implementation and a clear strategy, the potential benefits they offer position them as invaluable tools for any organization looking to thrive in an increasingly data-driven world.
How Machine Learning is Revolutionizing Business and Industry?
ML systems are transforming the landscape of healthcare by enhancing the predictive capabilities of medical professionals. With the power of advanced algorithms, they can identify potential health risks earlier, enabling proactive measures rather than reactive treatments. These systems also have a significant impact in drug discovery, accelerating the pace at which new medications reach the market by predicting their efficacy and safety profiles more efficiently.
In the financial sector, machine learning systems have revolutionized the way institutions approach data analysis and decision making. By employing these systems, banks and investment firms are able to process colossal volumes of transactions in real time, identifying fraudulent activities almost instantaneously and mitigating financial risks more effectively. Moreover, they provide bespoke investment solutions based on individual client data, reshaping the personalized banking experience.
Retailers have seen a profound transformation thanks to ML systems, as they now have the ability to understand and predict consumer purchasing behavior at an individual level. This insight enables retailers to offer highly targeted marketing campaigns, optimize their stock levels, and improve customer service by anticipating needs. Machine learning algorithms also streamline logistics, reducing waste and inefficiencies, which ultimately contributes to a more sustainably conscious approach to business operations.
In the manufacturing sector, the integration of machine learning systems has brought a new level of sophistication to production lines. By employing sophisticated pattern recognition and analysis, these systems can predict machine failures before they occur, scheduling maintenance only when necessary rather than following a fixed schedule. This not only cuts downtime but also extends the lifespan of machinery. Further, with the use of machine learning, manufacturers can quickly adapt to changes in demand, optimizing production schedules and reducing overproduction, leading to smarter and more responsive manufacturing processes.
What is the difference between AI and machine learning?
Artificial Intelligence (AI) is a broader concept that refers to machines designed to perform tasks that typically require human intelligence. Meanwhile, machine learning is a subset of AI that focuses on the idea that systems can learn from data, identify patterns, and make decisions with minimal human intervention. Essentially, all machine learning is AI, but not all AI is machine learning.
To further grasp the distinction, it’s important to understand that AI encompasses a wide range of technologies, including robotics, natural language processing, and expert systems. These technologies may not necessarily involve learning from data, but rather operate based on predefined rules and logic. On the other hand, machine learning systems specifically rely on algorithms that enable them to evolve as they process more data, hence they are not bound by static programming directives.
Furthermore, AI aims to simulate human intelligence broadly, sometimes by attempting to replicate our cognitive processes. In contrast, ML systems do not strive to mimic human thought. Instead, they discern patterns and correlations within datasets to generate predictions or categorize information, activities that are typically only one component of human intelligence. Recognizing this distinction is vital for leveraging the right type of computational technology for the right task within innovation and business processes.
What are the 4 types of machine learning?
When delving into ML systems, it becomes apparent that there are distinct approaches these machine learning systems may adopt, with each method suited to particular types of problems. Supervised learning stands out as the most prevalent type, relying on input-output pairs that serve as explicit examples for the system to learn from. This training on labeled data is akin to a teaching process where the desired outcome is known, and the algorithm incrementally improves its predictions over time.
Unsupervised learning, by contrast, does not work with predefined labels. Instead, it analyzes data to uncover hidden structures or patterns without previous human categorization. This type of learning is particularly useful for exploratory data analysis, clustering, and association tasks where the structures within the data are not yet known.
There’s also semi-supervised learning, which exists somewhere in between supervised and unsupervised learning. It utilizes a smaller amount of labeled data augmented with a larger set of unlabeled data. This amalgamation allows machine learning models to benefit from the large amount of data available while still guiding the learning process with some human insight.
Lastly, reinforcement learning is a unique type wherein an agent learns to make decisions through trial and error to achieve a certain goal. This method involves interaction with an environment and is guided by rewards or penalties that signal the agent’s performance. It is frequently utilized in scenarios where real-time decision making is paramount, such as in robotic controls or in game play scenarios.
Each type of machine learning can tackle diverse challenges and is selected based on the nature of the problem and the data available. Understanding these types is crucial for anyone working with or relying on ML systems to support decision-making and strategic planning within their organizations.
What is the risk in using ML?
One of the key risks in using ML systems is the potential for bias in the data, leading to skewed results and unfair decision-making. Moreover, there could be a lack of transparency in machine decision processes, making it challenging for stakeholders to understand how results are derived. Data privacy and security concerns are also paramount, as machine learning systems require access to large amounts of sensitive information.
ML systems also face challenges related to overfitting, where a model performs well on training data but fails to generalize to new data. This can lead to poor performance and unreliable predictions when the model encounters data from real-world scenarios. Furthermore, the complexity and sophistication of these systems can result in costly and time-consuming development, requiring expert knowledge to design, implement, and maintain.
Another significant risk involves the reliance on algorithms that may perpetuate existing prejudices found in training data. Without careful consideration and regular assessments, machine learning systems might automate and amplify biases, which can have profound negative impacts on individuals and society. In addition, there are concerns regarding the accountability when decisions are made by machines rather than humans, particularly in critical sectors such as healthcare and law enforcement.
How can ML help companies to gain awareness?
Machine learning systems can be instrumental for companies looking to gain insights into market trends, consumer behaviors, and operational inefficiencies. By analyzing data at a scale and speed beyond human capabilities, ML provides valuable insights that can inform strategic decisions and create competitive advantages.
ML systems aid in demystifying complex market dynamics by detecting subtle patterns and shifts that might not be immediately apparent. This advanced analysis enables organizations to adapt quickly to changing market conditions, predict consumer needs, and adjust their strategies accordingly. As a result, businesses that effectively deploy machine learning capabilities can stay ahead of the curve and respond proactively to emerging opportunities.
Furthermore, the integration of machine learning systems into corporate strategies allows for the improvement of customer engagement and satisfaction. By sifting through customer data, ML can uncover preferences and behaviors, tailoring marketing efforts to individual needs. This level of personalization not only fosters brand loyalty but also streamlines customer acquisition and retention efforts. In essence, machine learning can transform vast amounts of data into actionable intelligence, propelling a company’s growth trajectory forward.
In addition to market intelligence, machine learning systems also play a pivotal role in internal awareness, equipping companies with the tools necessary to optimize operations. Through predictive analytics, organizations can anticipate and prevent potential issues in their supply chain or manufacturing processes. This proactive maintenance can result in significant cost savings and enhanced productivity, driving the overall efficiency of a company. Adopting ML as part of an operational strategy can thus act as a significant enabler, helping businesses to thrive in an ever-competitive landscape.
What are some limitations of machine learning?
Despite its transformative potential, machine learning has limitations. These include a reliance on high-quality, large datasets, and the difficulty in understanding complex ML models, commonly referred to as the “black box” problem. Additionally, machine learning can’t incorporate human nuances such as ethical considerations or common sense reasoning, which may be crucial in some decision-making scenarios.
Another significant challenge is that machine learning systems require extensive computational resources. Training complex models necessitates a substantial amount of processing power and memory, which can be a barrier for smaller organizations with limited technological infrastructure. This computational demand also leads to higher energy consumption, posing environmental concerns and increasing operational costs.
Furthermore, the effectiveness of ML systems largely depends on the quality of data input. Poorly curated data can result in inaccurate outcomes, as the system is only as good as the information it learns from. This reliance on data quality means that any errors or anomalies in the data set can severely impact the results, leading to flawed decision-making or misguided efforts that can stifle innovation and progress in businesses and industries.
Conclusion
Machine learning (ML) systems are rapidly transforming the business landscape, creating in a new era of automation, efficiency, and data-driven decision-making. By leveraging the power of advanced algorithms, businesses across diverse industries are gaining a significant competitive edge. From streamlining operations to predicting customer behavior and identifying emerging trends, ML offers a comprehensive suite of tools to optimize performance and unlock new possibilities.
While challenges like data bias and algorithmic complexity remain, the potential benefits of ML are undeniable. As these technologies continue to evolve, businesses that embrace ML will be well-positioned to thrive in the dynamic and ever-changing world of tomorrow. The future of business is undoubtedly intertwined with the advancements in machine learning, and those who actively implement these solutions will be the ones shaping the course of the industry.